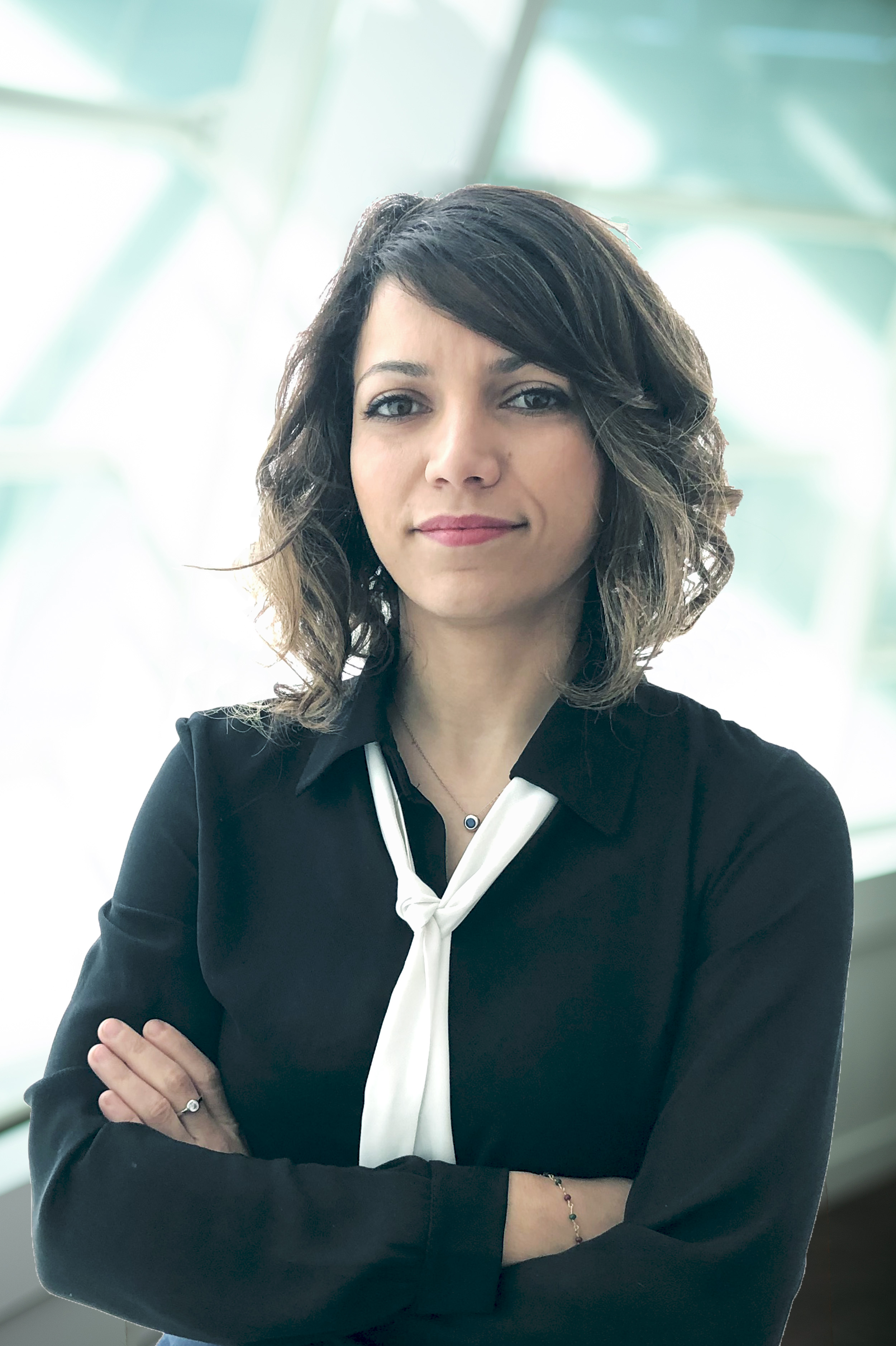
Can you tell us a bit about your career, your background and what projects you have worked on in the past?
I am the Head of Data Science working in the Data & Transformation department at BNP Paribas Real Estate. I graduated in Operational Research Engineering and have a Master’s degree in Applied Mathematics from the Paris Dauphine University.
I started my career as a Research & Development Engineer, working within the transportation sector. During this time I mostly worked on analysing and forecasting traffic which was related to public and private transport.
I then took on a job working as a Data Scientist at an e-commerce start-up and after spent three years as a Consultant Data Scientist within the banking sector, during which time I mainly worked for the BNP Paribas Group in France and abroad. In this role, my main focus was working with the different business lines in order to identify business issues and cases, collecting and analysing large amounts of data and building models using advanced analytics techniques (clustering, predictions, scoring etc.). The results of these models were then shared with the business lines in order to give them a vision of their clients and how services for them could be offered, in line with what their original business case had been.
I started at BNP Paribas Real Estate in September 2018, heading up the Data Science team.
What is data science exactly?
For me the clearest definition of data science is one that is found on many websites, which is that data science is ‘the art of uncovering insight and extracting knowledge from data’.
The concept of data science is not something that is new, computer scientists and mathematicians have long been studying data. What is new is the coining of the job title Data Scientist which defines the process of making sense of the huge amounts of data that we can now have at our fingertips.
The data science field comprises mathematics, statistics and computer science disciplines, and incorporates techniques like machine learning, deep learning, cluster analysis, data mining and visualisation.
With data science allowing businesses to understand trends and different behaviour types in relation to their clients, it is unsurprising that businesses from a whole range of sectors have been keen to develop their data science capabilities. As a result of machine learning for example, TV streaming services are truly able to analyse the behaviour of their users. Using user’s search history, the age and sex categories that they fit into, the similarities between videos that they watch and those available and comments left by subscribers, TV streaming sites are able to deduce what their subscribers are interested in and offer them targeted and relevant suggestions.
In terms of online shopping, having enough information which corresponds to that of another user (age, sex, location, products bought etc.) means that online shopping websites can offer users products which have been bought by other users that they might not have seen.
In what form does this data come in?
Today, we have a wealth of data available to us. This data can be presented in different forms including ‘structured’ data, meaning it is highly organised and means searching for specific entries is straight forward, as would be found in a database for example. Other types include ‘semi-structured’ and ‘unstructured’ which is where data is not organised in a structured format but machine learning can allow the data to be separated or organised, this might be data in emails or photos for example.
Data that is available in these forms exists in huge volumes and what data science allows is the processing and analysing of this data in order to identify patterns that can help with strategic decision making, increase business efficiency and optimise certain processes.
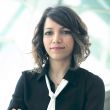
![]()
In the near future, data science will have an important role to play, as it will be able to not only improve a business strategy but also improve the way and quality of our lives.
What is the role of a Data Scientist? What kind of skills do they need to have?
The work of a Data Scientist has a lot of interesting aspects and a lot of challenges. To carry out such a job a Data Scientist should be proficient in maths, have great computer science skills, be curious and have excellent communication abilities.
In their day-to-day job a Data Scientist will need to spend a lot time with the different teams within a business in order to ask questions and identify relevant issues that need to be resolved. They therefore need to have a great understanding of the business and how data science contributes to that particular field.
Once a business case is identified, the Data Scientist will be tasked with collecting data from a multitude of different data sources (business data, API, customer reviews, videos, social media posts etc.). They will then need to clean and transform this data in order to make it usable.
After this process, a crucial part will be exploratory data analysis, which combines visualisation and statistics. This analysis aims to both define and refine the selection of variables that will be used in the model that a Data Scientist will develop.
The next step will be choosing one or more potential models and algorithms that fit the business’ requirements. The Data Scientist will apply advanced statistical models, machine learning and deep learning techniques, and will evaluate the results (measure the performance of the model, improve the result).
Finally, they will communicate their findings to the stakeholders in order to demonstrate how the results of the model can be used to improve the business aims.
What is the history of data science in the real estate sector?
In terms of the real estate sector, in America the use of data science is at a point where it is very developed. Real estate companies are investing in property matching online software, which can determine if a property is a good match in terms of investment for each customer. Models that allow this have been developed by using a variety of public data and market information such as prices per square metre on past transactions, number of bedrooms and the quality of the neighbourhood. This provides clients with much wider parameters than could be provided by a single estate agent and allows the estate agent to offer greater accuracy in their information, for by example, giving clients accurate house prices instead of estimations. Zillow, an American real estate company, has developed a ‘Zestimate’ prediction, which predicts what the value of a property currently on sale will be anytime for one to ten years from now.
What makes data science in the real estate sector different to other sectors?
The real estate sector is a sector whose reach is vast, it is therefore affected by many social, political and economic factors which means that there is a huge amount of complex data available. It is through analysing and understanding this data that models can be created which aim to replicate the changes in the sector and evolve in order to anticipate what we might see in the future.
For example by analysing market trends, models can help clients optimise the purchase of a property or even help to signal a potential market crisis such as the subprime mortgage crisis of 2008.
Where do you look to acquire data? How can a Data Scientist extract data from a building or piece of real estate?
Sources of data are very diverse: open source data, transactional data or data which comes directly from business lines to name a few.
Data from a building can be extracted through status reports but also using new technology such as the Internet of Things (IoT), which can monitor temperature and the condition of equipment. This combination of sources provides information on the daily use of the building. What is also important is collecting data from the building’s environment, such as data related to urbanism, localisation, demography and neighbourhoods. Doing this means through the data, a Data Scientist is able to a have a fully rounded vision of a building, its environment and its users.
The success of data science in real estate is however dependent on the quality of the data and how it can be accessed. The volume available is huge, sources are multiple and not always in line with one another. A huge amount of data sits in paper files or PDF documents, so it’s not easy to access it even though very relevant. A key challenge during these first stages of data science in real estate is therefore understanding how to structure and qualify data in order to eliminate non-relevant or unreliable sources and focus on dependable data that is available for use.
What role do you believe data science will play in the future of the real estate sector?
In the near future, data science will have an important role to play, as it will be able to not only improve a business strategy but also improve the way and quality of our lives. Data science which works in tandem with Artificial Intelligence (AI) will be able to analyse behaviour, interests and preferences in order to propose the ideal apartment for each client. This will mean that clients interested in a property will be able to visit it on their smartphone, projecting themselves into what it would be like to live there, by eliminating a wall or changing the colours for example.
Users installed within a building will also benefit from greater understanding of data. The IoT is becoming more and more necessary and used in AI within the real estate sector. Sensors which record temperature, air, equipment condition allow for responsive environments which adapt to user’s habits and behaviour. Data will thus have a direct impact on the chosen place of residence and investment criteria and will increasingly influence buying and selling habits.
All of this is done while respecting the European Regulation (GDPR and others) so the data remains unobtrusive but intuitive to users and businesses.

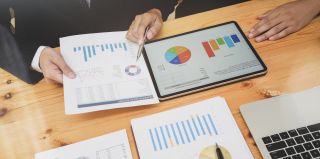
Learn more from our publication
BNP Paribas Real Estate provides in-depth documents to give you an overview of the major trends affecting the European real estate market.